AI Business Framework and Maturity Model
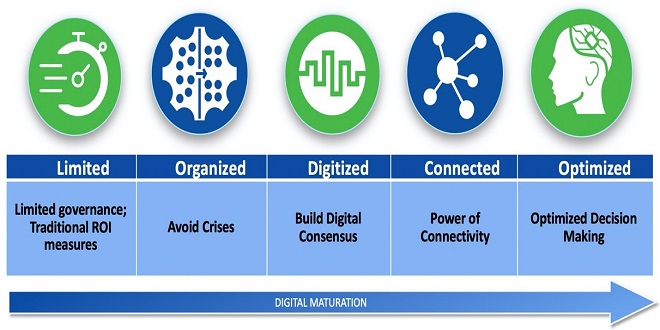
Methods and Technologies
In the following, the various methods and technologies are brief outlined and explained.
Since the conference at Dartmouth College in 1956, a variety of different methods and technologies have been developed for the construction of intelligent systems. Even if neuronal networks and thus the approach of sub-symbolic AI dominates today, the Feld of research was dominated by the symbolic approach for a long time. Tis “classical” approach by John Hoagland called “Good Old-Fashioned Artificial Intelligence” (GOFAI) used defend rules to come to intelligent conclusions depending on the input. Up to the AI winter of the 1990s, “artificial intelligences” were developed by programming and fling control equipment and standards and databases to then be able to access them in practice.
Natural Language Processing (NLP)
Computer linguistics covers the understanding, processing and generating of languages. “Natural language processing” describes the ability computers have to work with spoken or written text by extracting the meaning from the text or even generating text that is readable, stylistically natural and grammatically correct. With the help of NLP systems, computers are put in a position of not only reacting to formalized computer languages such as Java or C, but also to natural languages such as German or English.
Rule-Based Expert Systems
Rule-based expert systems belong to one of the first profitable implementations of AI that are applied to this day. Felds of use are multifaceted and range from planning in logistics and air traffic over the production of consumer and capital goods down to medical diagnostics systems. They are distinguished by the fact that the knowledge represented inside of them originates from experts (individual Felds of expertise) in its nature and origin. Depending on the input variables, automatic conclusions are then derived from this knowledge. To this end, the knowledge (in the spirit of symbolic AI) must be codified, i.e. furnished with rules, and be linked to a derivation system to solve the challenges.
Sub-symbolic AI
Te approach of symbolic AI to systematically capture and codify knowledge was considered very promising for a long time. In a world that is being digitalised further and further, in which knowledge implicitly lies in the amounts of data, AI should be able to do something that knowledge-based expert systems inherently fnddifcult: Self-learning. Deep Blue, for example, was in fact able to beat Garry Kasparow in 1996 without the use of artificial neuronal networks, but only because the chess game had been formalized by humans and because the computer was able to compute up to 200 million moves per second from which the most promising one was then chosen.
Machine Learning
The term machine learning (ML) as a part of artificial intelligence is ubiquitous nowadays. The term is used for a wide number of various applications and methods that deal with the “generation of knowledge from experience”. The well-known US computer scientist Tom Mitchell defines machine learning as follows: A computer program is said to learn from experience E with respect to some class of tasks T and performance measure P, if its performance at tasks in T, as measured by P, improves with experience E (Mitchell 1997). An illustrative example of this would be a chess computer program that improves its performance (P) in playing chess (the task T) by experience (E), by playing as many games as possible (even against itself) and analyzing them (Mitchell 1997).
Supervised Learning
Supervised learning proceeds within clearly defend limits. Besides the actual data set, the right possible answers are already known. The supervised learning methods are meant to reveal the relationship between input and output data. These methods are used for tasks in the Felds of classification as well as regression analyses. Regression is about predicting the results within a continuous output, which means that an attempt is made to allocate input variables to a continuous function. With the classification, in contrast, an attempt is made to predict results in a discreet output, i.e. allocate input variables to discreet categories.
Lastly Comment
Whilst with the automated enterprise the approaches of narrow AI described in are applied, the super intelligence enterprise concludes the potential of autonomy and self-learning of companies by way of general and super intelligence. Tis scenario currently appearing to be hardly realistic has two types of manifestation. In the positive version, we as humans control the framework conditions and rules of the autonomous AI systems. We can intervene and rectify via regulative and corrective measures at any time. Productivity and well-being are increased further by the performance, scalability and innovations of these super intelligences. In the negative version, we as humans have lost control over the framework conditions and rules of the autonomous systems.